TinyML triages and prioritizes Covid-19 patients in Emergency Departments
Despite the fact that we are in the middle of a significant transformation regarding the way products and services are produced and distributed, known as Industry 4.0, Health 4.0 is still in its infancy. However, new IoT technologies and techniques are making the difference aiming to change the way healthcare is delivered. The recent Covid-19 outbreak highlighted the weaknesses of healthcare systems across the world and revealed the clinical need for new innovative IoT solutions.
In order to limit the spread of Covid-19, certain measures are needed to alleviate the burden from public health organisations, including reference hospitals. One of the most significant is to reduce Emergency Department (ED) overcrowding, minimising waiting time of suspicious incidents and increase in-hospital safety. Fever and shortness of breath are considered as two of the main symptoms of patients infected by Covid-19. Both symptoms can be objectively tracked by measuring vital signs, such as body temperature, breathing rate and/or oxygen saturation levels. Continuous monitoring of those vitals is not currently considered as an option offered by EDs, unless a patient is admitted to the ICU. Instead, sporadic checks may occur depending on the availability of human resources (usually limited and under huge pressure). In addition, the commercially available medical devices are not always portable, while more than one device is needed to provide the full spectrum of required vital signs.
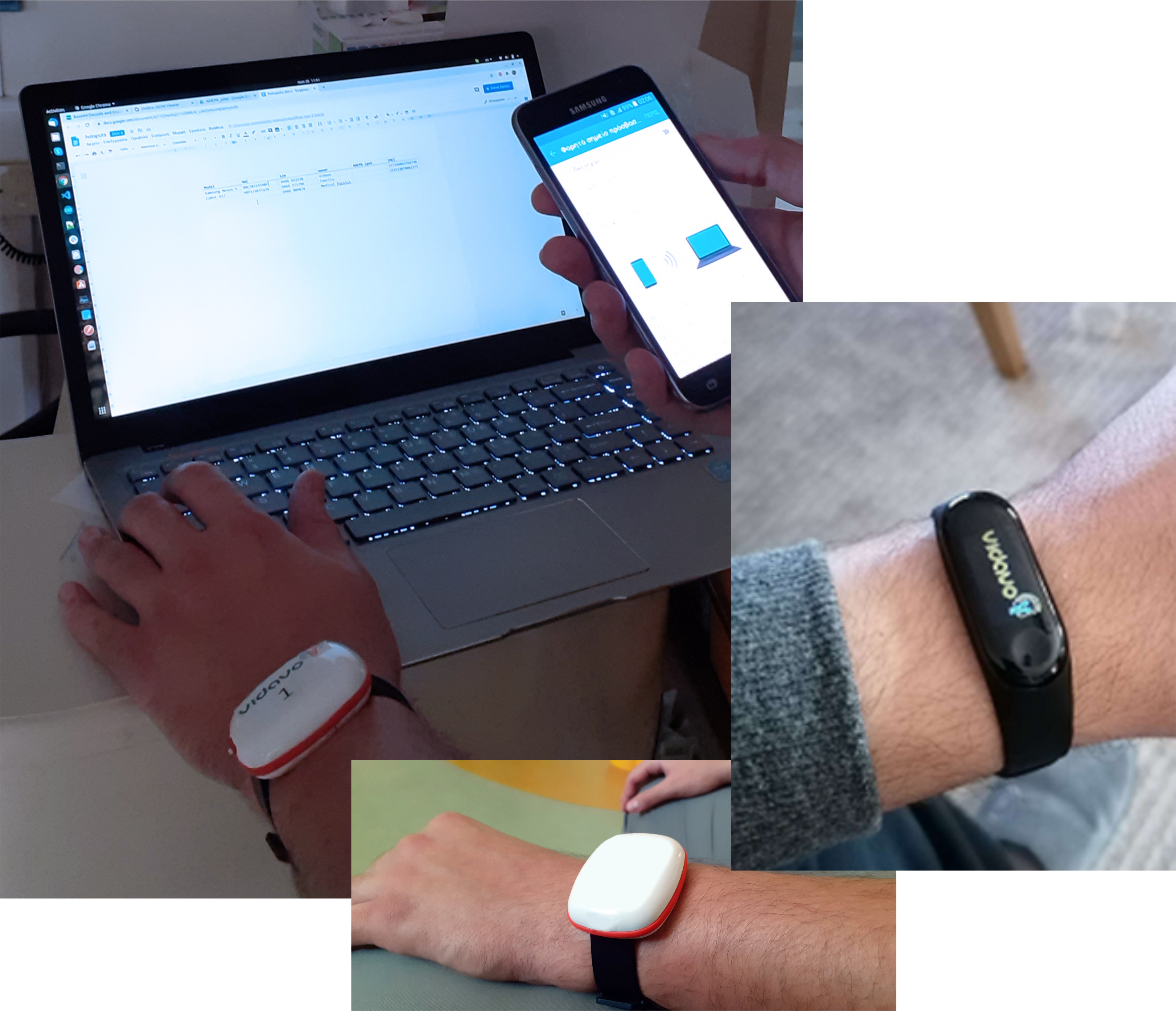
Exploiting edge computing and advanced digital signal processing techniques, Vidavo designed and developed a wrist-wearable device able to continuously extract heart rate, blood oxygen saturation, body temperature and evaluates patients’ respiration. A significant novelty of the proposed solution is the use ML algorithms for respiration evaluation. Specifically, our team has designed and developed a machine learning model using Neural Networks (NNs) that can be run locally on the wearable device in order to avoid transmitting raw data over a radio channel to expensive, power hungry and vulnerable cloud infrastructures, ensuring data privacy, security and minimizing latency. The NN identifies three main patterns regarding respiration and deploys them on the wearable device in order to access and classify respiration patterns locally and offline highlighting the advantages of edge computing. The NN was designed and build using Edge Impulse, a user-friendly web application in order to execute the whole toolchain of a neural network, from raw data acquisition and extracting features to training models and deploy them on embedded devices.
The wearable device has been already interconnected with Vida24©, which is an integrated care solution, connecting smart medical devices, patients and medical experts already in use by thousands of EU citizens. Vida24© is certified CE0653 Class IIa medical software according to the EU Medical Devices Regulation (MDR 2017/745) and GDPR compliant.
The wrist-wearable device supports common communication protocols, thus being able to transfer processed data to other third-party systems and apps through an API. A mobile application for the ED personnel is used for real-time detection of health deterioration and enables highlighting the most critical Covid-19 cases, thus reducing clinical decision-making and patient waiting time and allowing for more efficient triage. Finally, recorded vital signs might act as a complementary source of information to the results of the clinical examination to make a final diagnosis of patients.
Working with our partners from Medical Physics lab from Medical School of Aristotle University of Thessaloniki, the proposed solution has been set up and piloted in the ED of AHEPA hospital in Thessaloniki, Greece, where suspicious Covid-19 cases are referred to from the Region of Central Macedonia.
The paper was published on IEEE GLOBECOM 2020 Special Workshop on Communications and Networking Technologies for Responding to COVID-19 in Taipei, Taiwan on December 2020. You can download the paper from IEEEXplore®.
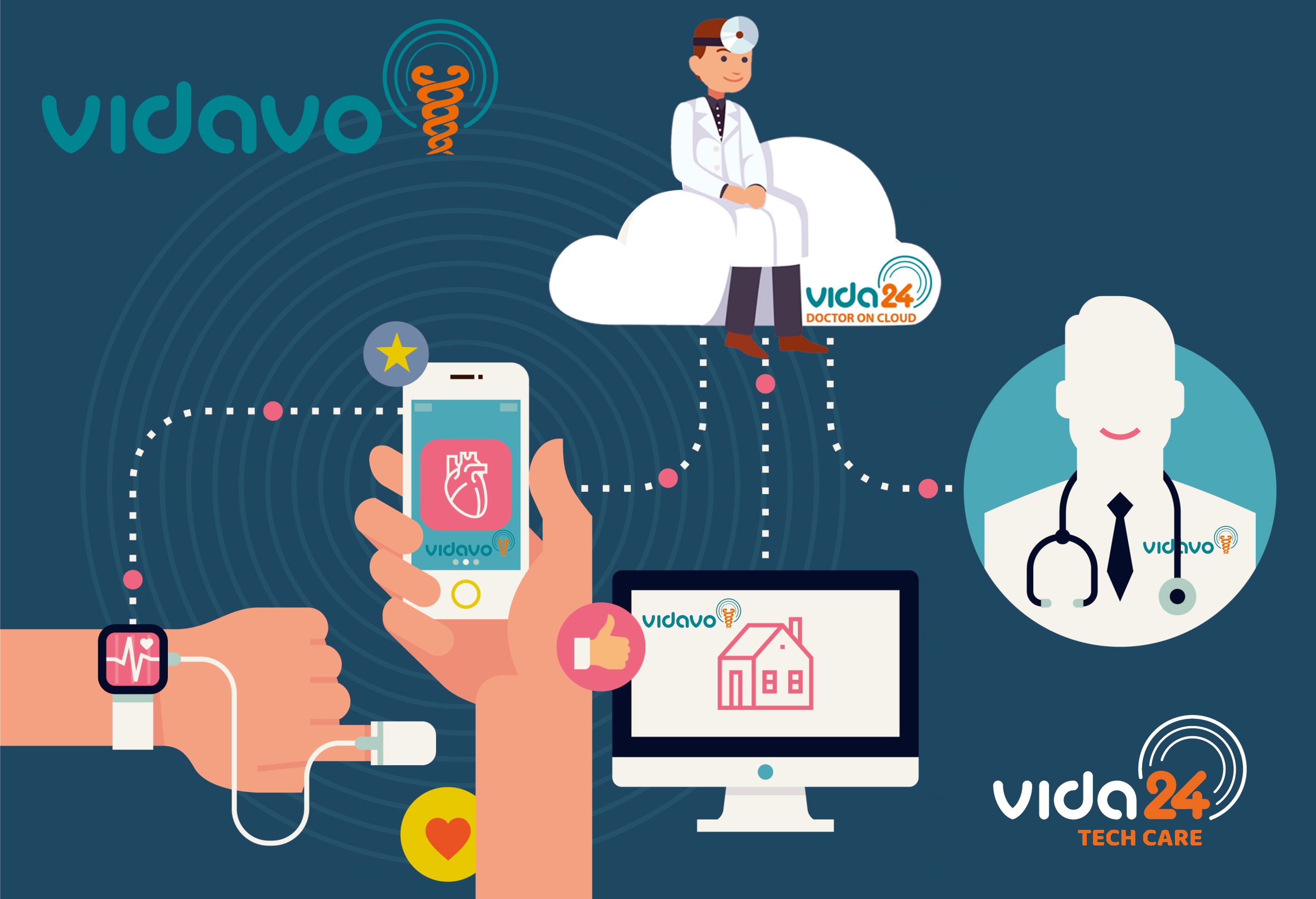